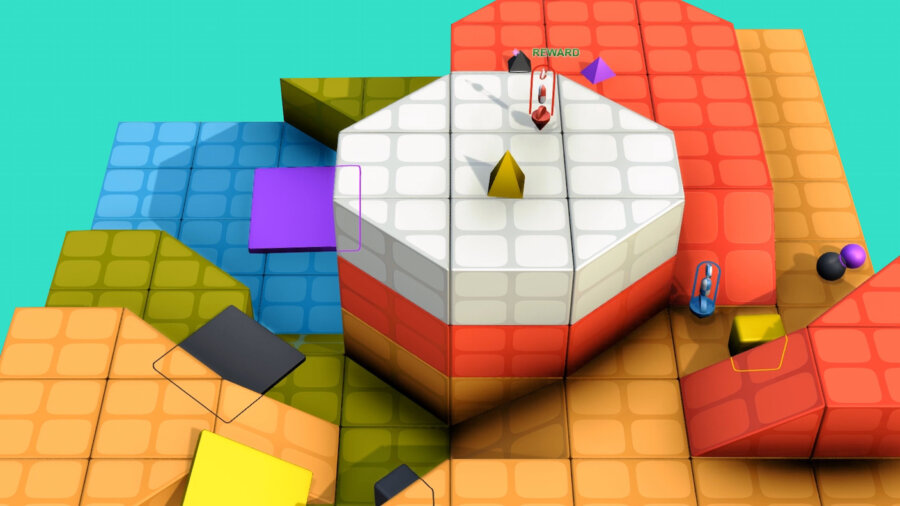
Last year, DeepMind researchers wrote that future AI developers may spend less time programming algorithms and more time generating rich virtual worlds in which to train them.
In a new paper released this week on the preprint server arXiv, it would seem they’re taking the latter part of that prediction very seriously.
The paper’s authors said they’ve created an endlessly challenging virtual playground for AI. The world, called XLand, is a vibrant video game managed by an AI overlord and populated by algorithms that must learn the skills to navigate it.
The game-managing AI keeps an eye on what the game-playing algorithms are learning and automatically generates new worlds, games, and tasks to continuously confront them with new experiences.
The team said some veteran algorithms faced 3.4 million unique tasks while playing around 700,000 games in 4,000 XLand worlds. But most notably, they developed a general skillset not related to any one game, but useful in all of them.
These skills included experimentation, simple tool use, and cooperation with other players. General skills in hand, the algorithms performed well when confronted with new games, including more complex ones, such as capture the flag, hide and seek, and tag.
This, the authors say, is a step towards solving a major challenge in deep learning. Most algorithms trained to accomplish a specific task—like, in DeepMind’s case, to win at games such as Go or Starcraft—are savants. They’re superhuman at the one task they know and useless at the rest. They can defeat world champions at Go or chess, but have to be retrained from scratch to do anything else.
By presenting deep reinforcement learning algorithms with an open-ended, always-shifting world to learn from, DeepMind says their algorithms are beginning to demonstrate “zero-shot” learning at new never-before-seen tasks. That is, they don’t need retraining to perform novel tasks at a decent level—sight-unseen.
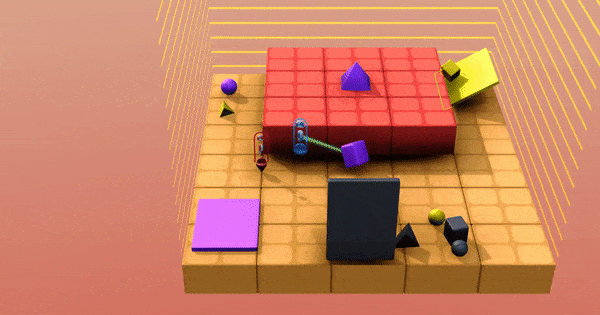
This is a step towards more generally capable algorithms that can interact, navigate, and solve problems in the also-endlessly-novel real world.
But XLand isn’t the AI community’s first inkling of generalization of late.
OpenAI’s GPT-3 can generate uncanny written passages—its primary purpose—but it can do other things too, like simple arithmetic and programming. And it can be fine-tuned with just a few examples. (OpenAI says GPT-3 demonstrates “few-shot” learning.)
And last year, DeepMind itself developed an algorithm that wrote a key piece of its own code called the value function, which guides its actions by projecting rewards. Surprisingly, after being trained in very simple “toy worlds,” the algorithm went on to play 14 Atari games it had never encountered at a superhuman level, performing, at times, on par with human-designed AI.
Notably, the more “toy worlds” the algorithm trained on, the better it could generalize. At the time, the team speculated that with enough well-designed training worlds, the approach might yield a general-purpose reinforcement learning algorithm.
XLand’s open-ended learning moves us further down that road. How far the road goes, however, is an open and hotly debated question.
Here, the algorithms are playing rather simple games in a relatively simple world (albeit cleverly tuned to keep things fresh). It isn’t clear how well the algorithms would do on more complex games, let alone in the world at large. But if XLand is a proof-of-concept, their findings may suggest increasingly sophisticated worlds will give birth to increasingly sophisticated algorithms.
Indeed, researchers at DeepMind recently put a stake in the ground, arguing (philosophically, at least) that reinforcement learning—the method behind the organization’s most spectacular successes—is all we need to get to artificial general intelligence. OpenAI and others, meanwhile, are going after unsupervised deep learning at scale for advanced natural language processing and image generation.
Not everyone agrees. Some believe deep learning will hit a wall and have to pair up with other approaches, like symbolic AI. But three of the field’s pioneers—Geoffrey Hinton, Yoshua Bengio, and Yann LeCun—recently co-wrote a paper arguing the opposite. They acknowledge deep learning’s shortcomings, including its lack of flexibility and inefficiency, but believe it will overcome its challenges without resorting to other disciplines.
Philosophical arguments aside, narrow AI is already having a big impact.
DeepMind showed as much recently with its AlphaFold algorithm, which predicts the shapes of proteins. The organization just released the predicted shapes of 350,000 proteins, including nearly every protein in the human body. They said another 100 million are on the way.
To put that in perspective, scientists have worked out the structure of some 180,000 proteins over decades. DeepMind’s protein drop nearly doubled the count in one fell swoop. The newly minted protein library hasn’t been rigorously confirmed by scientists, but it will be a valuable tool for them. Instead of starting from a blank slate, they’ll have a template (perhaps much more) to work from.
Whatever becomes of the quest for artificial general intelligence, it seems there’s still plenty of room to run for its more vocational forerunners.
Image Credit: DeepMind
* This article was originally published at Singularity Hub
1 Comments
Post Which you have shared here. This post is very informative and I liked to see it. animal toys
ReplyDelete