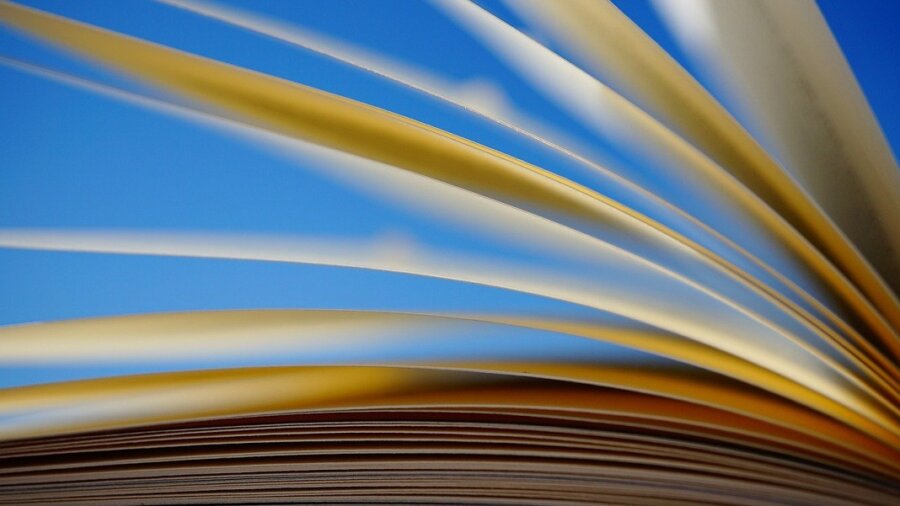
Just under a year and a half ago OpenAI announced completion of GPT-3, its natural language processing algorithm that was, at the time, the largest and most complex model of its type. This week, Microsoft and Nvidia introduced a new model they’re calling “the world’s largest and most powerful generative language model.” The Megatron-Turing Natural Language Generation model (MT-NLG) is more than triple the size of GPT-3 at 530 billion parameters.
GPT-3’s 175 billion parameters was already a lot; its predecessor, GPT-2, had a mere 1.5 billion parameters, and Microsoft’s Turing Natural Language Generation model, released in February 2020, had 17 billion.
A parameter is an attribute a machine learning model defines based on its training data, and getting more of them requires upping the amount of data the model is trained on. It’s essentially learning to predict how likely it is that a given word will be preceded or followed by another word, and how much that likelihood changes based on other words in the sentence.
As you can imagine, getting to 530 billion parameters required quite a lot of input data and just as much computing power. The algorithm was trained using an Nvidia supercomputer made up of 560 servers, each holding 8 80-gigabyte GPUs. That’s 4,480 GPUs total, and an estimated cost of over $85 million.
For training data, Megatron-Turing’s creators used The Pile, a dataset put together by open-source language model research group Eleuther AI. Comprised of everything from PubMed to Wikipedia to Github, the dataset totals 825GB, broken down into 22 smaller datasets. Microsoft and Nvidia curated the dataset, selecting subsets they found to be “of the highest relative quality.” They added data from Common Crawl, a non-profit that scans the open web every month and downloads content from billions of HTML pages then makes it available in a special format for large-scale data mining. GPT-3 was also trained using Common Crawl data.
Microsoft’s blog post on Megatron-Turing says the algorithm is skilled at tasks like completion prediction, reading comprehension, commonsense reasoning, natural language inferences, and word sense disambiguation. But stay tuned—there will likely be more skills added to that list once the model starts being widely utilized.
GPT-3 turned out to have capabilities beyond what its creators anticipated, like writing code, doing math, translating between languages, and autocompleting images (oh, and writing a short film with a twist ending). This led some to speculate that GPT-3 might be the gateway to artificial general intelligence. But the algorithm’s variety of talents, while unexpected, still fell within the language domain (including programming languages), so that’s a bit of a stretch.
However, given the tricks GPT-3 had up its sleeve based on its 175 billion parameters, it’s intriguing to wonder what the Megatron-Turing model may surprise us with at 530 billion. The algorithm likely won’t be commercially available for some time, so it’ll be a while before we find out.
The new model’s creators, though, are highly optimistic. “We look forward to how MT-NLG will shape tomorrow’s products and motivate the community to push the boundaries of natural language processing even further,” they wrote in the blog post. “The journey is long and far from complete, but we are excited by what is possible and what lies ahead.”
Image Credit: Kranich17 from Pixabay
* This article was originally published at Singularity Hub
0 Comments