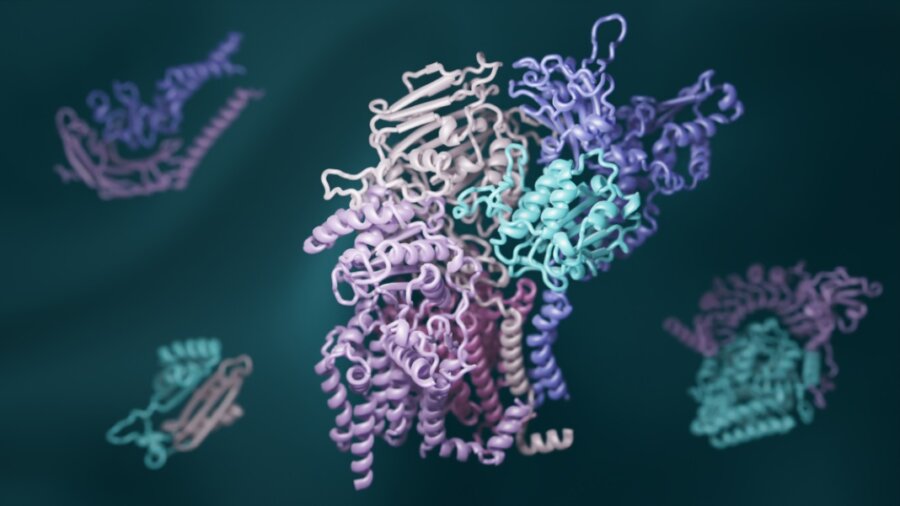
Thanks to deep learning, the central mysteries of structural biology are falling like dominos.
Just last year, DeepMind shocked the biomedical field with AlphaFold, an algorithm that predicts protein structures with jaw-dropping accuracy. The University of Washington (UW) soon unveiled RoseTTAFold, an AI that rivaled AlphaFold in predictive ability. A few weeks later, DeepMind released a near complete catalog of all protein structures in the human body.
Together, the teams didn’t just essentially solve a 50-year-old grand challenge in biology. Because proteins are at the heart of most of our medications, the programs unleashed a new era of drug development. For the first time, we have unprecedented insight into the protein engines of our cells, many of which had remained impervious to traditional lab techniques.
Yet one glaring aspect was missing. Proteins don’t operate alone. They often associate into complexes—small groups that interact to carry out critical tasks in our cells and bodies.
This month, the UW team upped the game. Tapping into both AlphaFold and RoseTTAFold, they tweaked the programs to predict which proteins are likely to tag-team and sketched up the resulting complex into a 3D model. Using AI, the team predicted hundreds of complexes—many of which are entirely new—that regulate DNA repair, govern the cell’s digestion system, and perform other critical biological functions. These under-the-hood insights could impact the next generation of DNA editors and spur new treatments for neurodegeneration disorders or anti-aging therapies.
“It’s a really cool result,” said Dr. Michael Snyder at Stanford University, who was not involved in the study, to Science.
Like a compass, the results guide experimental scientists to test out the predictions in search of new ideas into how our cells grow, age, die, malfunction, and reproduce. Several predictions further highlighted how our cells absorb external molecules—a powerful insight to coerce normally reluctant cells to gulp up medications. “It…gives you a lot of potential new drug targets,” said study author Dr. Qian Cong at the University of Texas Southwestern Medical Center.
The Cell’s Lego Blocks
Our bodies are governed by proteins, each of which intricately folds into 3D shapes. Like unique Lego bricks, these shapes allow the proteins to combine into larger structures, which in turn conduct biological processes that propel life.
Too abstract? An example: when cells live out their usual lifespan, they go through a process called apoptosis—in Greek, the falling of the leaves—in which the cell gently falls apart without disturbing its neighbors by leaking toxic chemicals. The entire process is a cascade of protein-protein interactions, whereby one protein grabs onto another to activate it, and subsequently releases it for the now activated protein to further stir up the next one in the chain, eventually causing the aging or diseased cell to sacrifice itself.
Another example: in neurons during learning, synapses (the hubs that connect brain cells) call upon a myriad of proteins that form a complex together. This, in turn, spurs the neuron’s DNA to make proteins that etch the new memory into the brain.
“Everything in biology works in complexes. So, knowing who works with who is critical,” said Snyder.
For decades, scientists have relied on painfully slow processes to parse out those interactions. One approach is computational: map out a protein’s structure down to the atomic level, and predict “hot spots” that might interact with another protein. Another is experimental: using both biological lab prowess and physics ingenuity, scientists can isolate protein complexes from cells—like sugar precipitating from lemonade when there’s too much of it—and use specialized equipment to analyze the proteins. It’s tiresome, expensive, and often plagued with errors.
Here Comes the Sun
Deep learning has now shined light on the whole enterprise.
The main idea is deceptively simple. Proteins are made of twisting strands and sheets of a single line of amino acids. Like beads strung onto a tangled but semi-predictable mess of yarn, deep learning can parse how those strings of yarn fold into 3D shapes based on the structure of the amino acid “beads” alone.
Last year, DeepMind and a team from UW led by Dr. David Baker both took a crack at the problem. Without knowing anything else about the protein, the programs were able to churn out thousands of protein structures. Though both were impressive, compared to AlphaFold2, Baker’s AI wasn’t as accurate for single protein predictions. But where RoseTTAFold shone was in predicting proteins with multiple sub-units—in essence, a single protein made up of a handful of structures, each physically grabbing onto the next. It’s a perfect jumping-off point for diving into protein handshakes.
At the time, the AI only worked on proteins in simple creatures, like bacteria. In the new study, Baker’s team focused on a more complicated organism—the common yeast, which has a cellular structure similar to that of humans. The choice of focusing on yeast proteins was deliberate: as a lab favorite, its genome is relatively small, and there’s a “gold standard” set of protein interactions to test out the updated algorithm.
Almost immediately, the team ran into problems. Compared to bacteria, which the older AI tackled, yeast had a far more complicated system for translating its DNA into proteins. Each step added noise. To get around the hiccup, the team used an evolutionary approach. If a protein-protein interaction is important for biology, they reasoned, then the “hands”—the protein interface—where they grab onto each other should change together as species evolve to maintain the interaction.
They compared the amino acid sequence—20 “letters” total, compared to DNA’s 4—of over 6,000 yeast proteins to nearly 6,500 other similar species. Like cracking a cipher, this allowed the team to hone in on the amino acids that change in lockstep. They then traced the “letters” to their protein owners and hypothesized that these owners likely formed a complex.
Using both AlphaFold and RoseTTAFold, the team next solved the 3D structure of these protein candidates. Surprisingly, each algorithm on its own struggled in performance and power consumption. But by tag-teaming, with RoseTTAFold screening protein pairs first, followed by AlphaFold, they achieved “excellent performance,” the team said, with a precision of 95 percent for the gold standard set.
They next expanded their test to over eight million co-evolved yeast protein pairs. Together, the new algorithm found over 1,500 pairs likely to interact, and drew up 3D models for about 800 that hadn’t previously been characterized—that is, about half.
The success rate is a triumph for biology. Digging deeper, the team found that most of the newly-predicted complexes and interactions “play roles in almost all key processes” and “provide broad insights into biological function.”
Among the AI-predicted complexes are those that control DNA repair after damage, a process dubbed homologous recombination. It’s a cellular machinery that CRISPR and its variants tap into. Understanding the protein members and complexes involved could potentially lead to new avenues for gene editing.
Other complexes are involved in the cell’s recycling mechanism, which often goes awry in diseases involving neurodegeneration. Over time, toxic proteins build up to overwhelm vulnerable neurons, exacerbating their malfunction. Other complexes include those needed for cells to swallow up nutrients and medication, those that unwind chromosomes—which house DNA—during reproduction, and those that make up factories that translate RNA into proteins.
Like any simulation, the results are only hypotheses for now. But they offer unprecedented clues, at a large scale, into potentially new complexes and functions that escaped previous ideas. These predictions are a great example of the promise of 3D structures, said Dr. John Jumper, one of the lead developers for AlphaFold. Just last month, his team at DeepMind posted a pre-print on AlphaFold-Multimer, an algorithmic variant that predicts protein complexes at about 67 percent accuracy in nearly 4,500 test cases.
The study is just the start. “As with any new method, it is important when interpreting the results to keep in mind the limitations of the approach,” the team warned. For example, the AI doesn’t work as well for protein complexes that only transiently interact, or those that have extremely complicated structures. The results are so far only tested in yeast protein complexes, and may miss those restricted to another species. The AI also isn’t very confident in its predictions—tests show confidence levels of nearly 70 percent for each complex.
But that’s the thrill. Thanks to deep learning, we’re cracking the complexes that run the gamut of biology at a massive scale. “It’s a really exciting time,” said Baker.
Image Credit: Ian C. Haydon / UW Medicine Institute for Protein Design
* This article was originally published at Singularity Hub
0 Comments