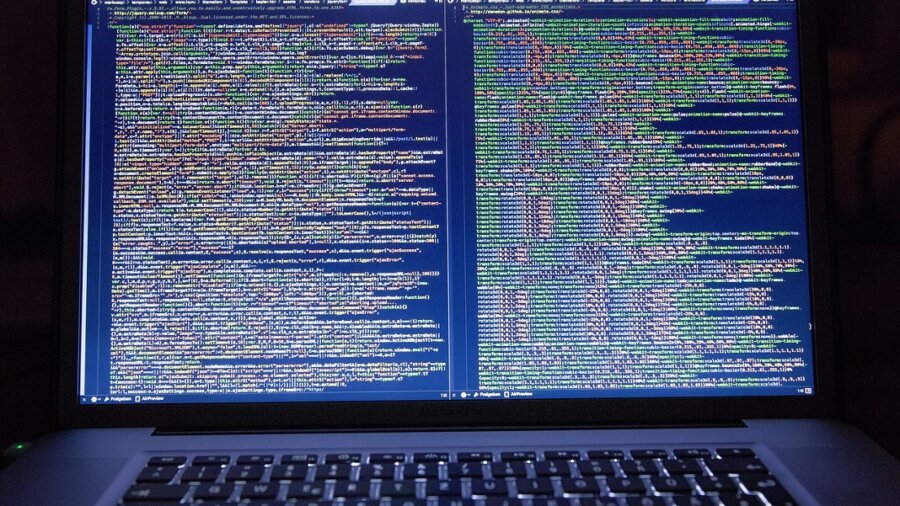
AI is exerting an ever greater influence on our lives, which is leading to growing concern over whether we can trust it to act fairly and reliably. Ethical hackers, AI audits, and “bias bounties” could help us keep a lid on the potential harms, say researchers.
There’s increasing awareness of the dangers posed by our reliance on AI. These systems have a worrying knack for picking up and replicating the biases already present in our society, which can entrench the marginalization of certain groups.
The data-heavy nature of current deep learning systems also raises privacy concerns, both due to their encouragement of widespread surveillance and the possibility of data breaches. And the black box nature of many AI systems also makes it hard to asses whether they’re working correctly, which can have serious implications in certain domains.
Recognition of these issues has led to a rapidly expanding collection of AI ethics principles from companies, governments, and even supranational organizations designed to guide the developers of AI technology. But concrete proposals for how to make sure everyone lives up to these ideals are much rarer.
Now, a new paper in Science proposes some tangible steps that the industry could take to increase trust in AI technology. A failure to do so could lead to a “tech-lash” that severely hampers progress in the field, say the researchers.
“Governments and the public need to be able to easily tell apart between the trustworthy, the snake-oil salesmen, and the clueless,” lead author Shahar Avin, from Cambridge University, said in a press release. “Once you can do that, there is a real incentive to be trustworthy. But while you can’t tell them apart, there is a lot of pressure to cut corners.”
The researchers borrow some tried and tested ideas from cybersecurity, which has grappled with the issue of getting people to trust software for decades. One popular approach is to use “red teams” of ethical hackers who attempt to find vulnerabilities in systems so that the designer can patch them before they’re released.
AI red teams already exist within large industry and government labs, the authors note, but they suggest that sharing experiences across organizations and domains could make this approach far more powerful and accessible to more AI developers.
Software companies also frequently offer “bug bounties,” which provide a financial reward if a hacker finds flaws in their systems and tells them about it privately so they can fix it. The authors suggest that AI developers should adopt similar practices, offering people rewards for finding out if their algorithms are biased or making incorrect decisions.
They point to a recent competition Twitter held that offered rewards to anyone who could find bias in their image-cropping algorithm as an early example of how this approach could work.
As cybersecurity attacks become more common, governments are increasingly mandating the reporting of data breaches and hacks. The authors suggest similar ideas could be applied to incidents where AI systems cause harm. While voluntary, anonymous sharing—such as that enabled by the AI Incident Database—is a useful starting point, they say this could become a regulatory requirement.
The world of finance also has some powerful tools for ensuring trust, most notably the idea of third-party audits. This involves granting an auditor access to restricted information so they can assess whether the owner’s public claims match their private records. Such an approach could be useful for AI developers who generally want to keep their data and algorithms secret.
Audits only work if the auditors can be trusted and there are meaningful consequences for a failure to pass them, though, say the authors. They are also only possible if developers follow common practices for documenting their development process and their system’s makeup and activities.
At present, guidelines for how to do this in AI are lacking, but early work on ethical frameworks, model documentation, and continuous monitoring of AI systems is a useful starting place.
The AI industry is also already working on approaches that could boost trust in the technology. Efforts to improve the explainability and interpretability of AI models are already underway, but common standards and tests that measure compliance to those standards would be useful additions to this field.
Similarly, privacy-preserving machine learning, which aims to better protect the data used to train models, is a booming area of research. But they’re still rarely put into practice by industry, so the authors recommend more support for these efforts to boost adoption.
Whether companies can really be prodded into taking concerted action on this problem is unclear. Without regulators breathing down their necks, many will be unwilling to take on the onerous level of attention and investment that these approaches are likely to require. But the authors warn that the industry needs to recognize the importance of public trust and give it due weight.
“Lives and livelihoods are ever more reliant on AI that is closed to scrutiny, and that is a recipe for a crisis of trust,” co-author Haydn Belfield, from Cambridge University, said in the press release. “It’s time for the industry to move beyond well-meaning ethical principles and implement real-world mechanisms to address this.”
Image Credit: markusspiske / 1000 images
* This article was originally published at Singularity Hub
0 Comments