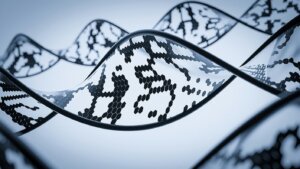
All life on Earth is written with four DNA “letters.” An AI just used those letters to dream up a completely new genome from scratch.
Called Evo, the AI was inspired by the large language models, or LLMs, underlying popular chatbots such as OpenAI’s ChatGPT and Anthropic’s Claude. These models have taken the world by storm for their prowess at generating human-like responses. From simple tasks, such as defining an obtuse word, to summarizing scientific papers or spewing verses fit for a rap battle, LLMs have entered our everyday lives.
If LLMs can master written languages—could they do the same for the language of life?
This month, a team from Stanford University and the Arc Institute put the theory to the test. Rather than training Evo on content scraped from the internet, they trained the AI on nearly three million genomes—amounting to billions of lines of genetic code—from various microbes and bacteria-infecting viruses.
Evo was better than previous AI models at predicting how mutations to genetic material—DNA and RNA—could alter function. The AI also got creative, dreaming up several new components for the gene editing tool, CRISPR. Even more impressively, the AI generated a genome more than a megabase long—roughly the size of some bacterial genomes.
“Overall, Evo represents a genomic foundation model,” wrote Christina Theodoris at the Gladstone Institute in San Francisco, who was not involved in the work.
Having learned the genomic vocabulary, algorithms like Evo could help scientists probe evolution, decipher our cells’ inner workings, tackle biological mysteries, and fast-track synthetic biology by designing complex new biomolecules.
The DNA Multiverse
Compared to the English alphabet’s 26 letters, DNA only has A, T, C, and G. These ‘letters’ are shorthand for the four molecules—adenine (A), thymine (T), cytosine (C), and guanine (G)— that, combined, spell out our genes. If LLMs can conquer languages and generate new prose, rewriting the genetic handbook with only four letters should be a piece of cake.
Not quite. Human language is organized into words, phrases, and punctuated into sentences to convey information. DNA, in contrast, is more continuous, and genetic components are complex. The same DNA letters carry “parallel threads of information,” wrote Theodoris.
The most familiar is DNA’s role as genetic carrier. A specific combination of three DNA letters, called a codon, encodes a protein building block. These are strung together into the proteins that make up our tissues, organs, and direct the inner workings of our cells.
But the same genetic sequence, depending on its structure, can also recruit the molecules needed to turn codons into proteins. And sometimes, the same DNA letters can turn one gene into different proteins depending on a cell’s health and environment or even turn the gene off.
In other words, DNA letters contain a wealth of information about the genome’s complexity. And any changes can jeopardize a protein’s function, resulting in genetic disease and other health problems. This makes it critical for AI to work at the resolution of single DNA letters.
But it’s hard for AI to capture multiple threads of information on a large scale by analyzing genetic letters alone, partially due to high computational costs. Like ancient Roman scripts, DNA is a continuum of letters without clear punctuation. So, it could be necessary to “read” whole strands to gain an overall picture of their structure and function—that is, to decipher meaning.
Previous attempts have “bundled” DNA letters into blocks—a bit like making artificial words. While easier to process, these methods disrupt the continuity of DNA, resulting in the retention of “ some threads of information at the expense of others,” wrote Theodoris.
Building Foundations
Evo addressed these problems head on. Its designers aimed to preserve all threads of information, while operating at single-DNA-letter resolution with lower computational costs.
The trick was to give Evo a broader context for any given chunk of the genome by leveraging a specific type of AI setup used in a family of algorithms called StripedHyena. Compared to GPT-4 and other AI models, StripedHyena is designed to be faster and more capable of processing large inputs—for example, long lengths of DNA. This broadened Evo’s so-called “search window,” allowing it to better find patterns across a larger genetic landscape.
The researchers then trained the AI on a database of nearly three million genomes from bacteria and viruses that infect bacteria, known as phages. It also learned from plasmids, circular bits of DNA often found in bacteria that transmit genetic information between microbes, spurring evolution and perpetuating antibiotic resistance.
Once trained, the team pitted Evo against other AI models to predict how mutations in a given genetic sequence might impact the sequence’s function, such as coding for proteins. Even though it was never told which genetic letters form codons, Evo outperformed an AI model explicitly trained to recognize protein-coding DNA letters on the task.
Remarkably, Evo also predicted the effect of mutations on a wide variety of RNA molecules—for example, those regulating gene expression, shuttling protein building blocks to the cell’s protein-making factory, and acting as enzymes to fine-tune protein function.
Evo seemed to have gained a “fundamental understanding of DNA grammar,” wrote Theodoris, making it a perfect tool to create “meaningful” new genetic code.
To test this, the team used the AI to design new versions of the gene editing tool CRISPR. The task is especially difficult as the system contains two elements that work together—a guide RNA molecule and a pair of protein “scissors” called Cas. Evo generated millions of potential Cas proteins and their accompanying guide RNA. The team picked 11 of the most promising combinations, synthesized them in the lab, and tested their activity in test tubes.
One stood out. A variant of Cas9, the AI-designed protein cleaved its DNA target when paired with its guide RNA partner. These designer biomolecules represent the “first examples” of codesign between proteins and DNA or RNA with a language model, wrote the team.
The team also asked Evo to generate a DNA sequence similar in length to some bacterial genomes and compared the results to natural genomes. The designer genome contained some essential genes for cell survival, but with myriad unnatural characteristics preventing it from being functional. This suggests the AI can only make a “blurry image” of a genome, one that contains key elements, but lacks finer-grained details, wrote the team.
Like other LLMs, Evo sometimes “hallucinates,” spewing CRISPR systems with no chance of working. Despite the problems, the AI suggests future LLMs could predict and generate genomes on a broader scale. The tool could also help scientists examine long-range genetic interactions in microbes and phages, potentially sparking insights into how we might rewire their genomes to produce biofuels, plastic-eating bugs, or medicines.
It’s yet unclear whether Evo could decipher or generate far longer genomes, like those in plants, animals, or humans. If the model can scale, however, it “would have tremendous diagnostic and therapeutic implications for disease,” wrote Theodoris.
Image Credit: Warren Umoh on Unsplash
* This article was originally published at Singularity Hub
0 Comments