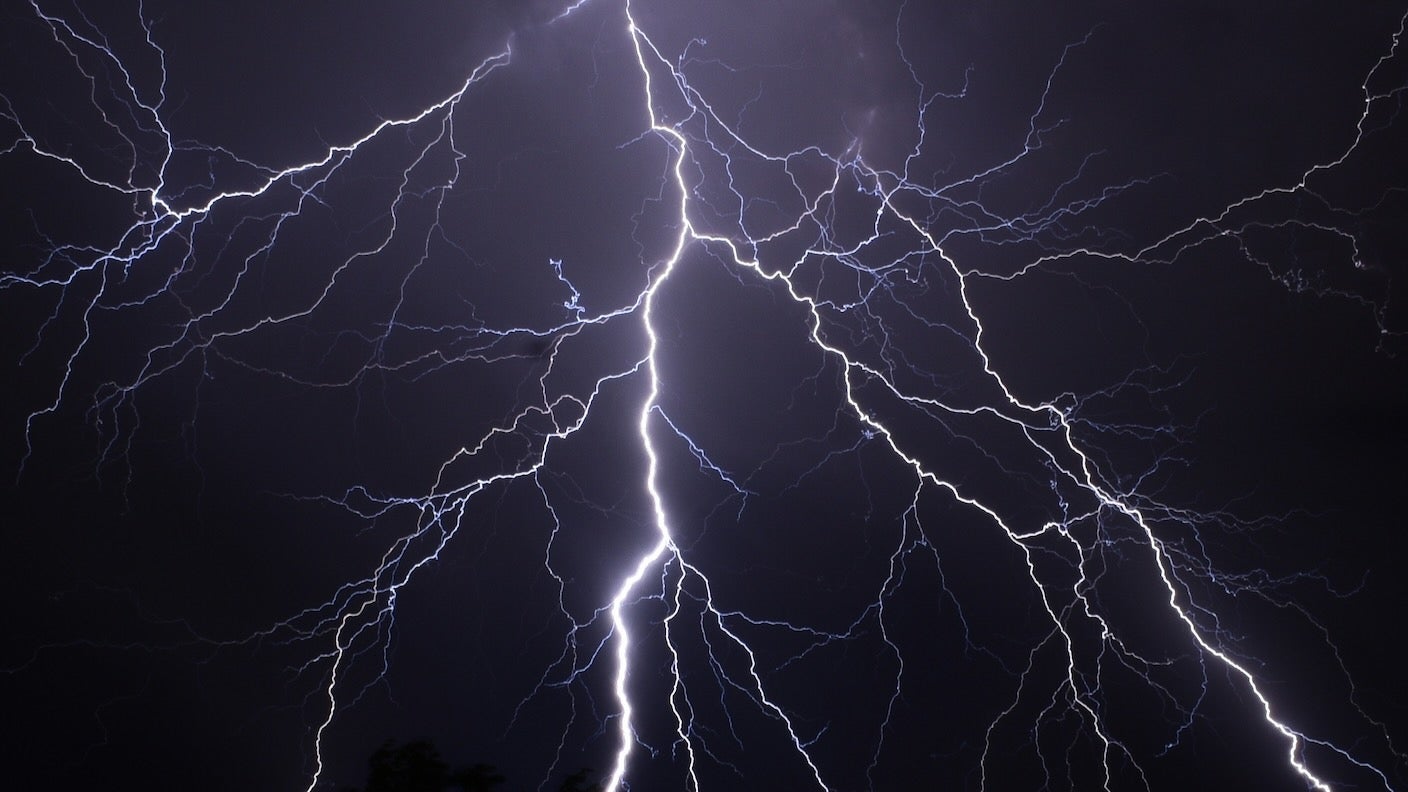
Inefficient AI models guzzle energy. Then again, so do efficient ones—just for different reasons.
DeepSeek has upended the AI industry, from the chips and money needed to train and run AI to the energy it’s expected to guzzle in the not-too-distant future. Energy stocks skyrocketed in 2024 on predictions of dramatic growth in electricity demand to power AI data centers, with shares of power generation companies Constellation Energy and Vistra reaching record highs.
And that wasn’t all. In one of the biggest deals in the US power industry’s history, Constellation acquired natural gas producer Calpine Energy for $16.4 billion, assuming demand for gas would grow as a generation source for AI. Meanwhile, nuclear power seemed poised for a renaissance. Google signed an agreement with Kairos Power to buy nuclear energy produced by small modular reactors (SMRs). Separately, Amazon made deals with three different SMR developers, and Microsoft and Constellation announced they would restart a reactor at Three Mile Island.
As this frenzy to secure reliable baseload power built towards a crescendo, DeepSeek’s R1 came along and unceremoniously crashed the party. Its creators say they trained the model using a fraction of the hardware and computing power of its predecessors. Energy stocks tumbled and shock waves reverberated through the energy and AI communities, as it suddenly seemed like all that effort to lock in new power sources was for naught.
But was such a dramatic market shake-up merited? What does DeepSeek really mean for the future of energy demand?
At this point, it’s too soon to draw definitive conclusions. However, various signs suggest the market’s knee-jerk response to DeepSeek was more reactionary than an accurate indicator of how R1 will impact energy demand.
Training vs. Inference
DeepSeek claimed it spent just $6 million to train its R1 model and used fewer (and less sophisticated) chips than the likes of OpenAI. There’s been much debate about what exactly these figures mean. The model does appear to include real improvements, but the associated costs may be higher than disclosed.
Even so, R1’s advances were enough to rattle markets. To see why, it’s worth digging into the nuts and bolts a bit.
First of all, it’s important to note that training a large language model is entirely different than using that same model to answer questions or generate content. Initially, training an AI is the process of feeding it massive amounts of data that it uses to learn patterns, draw connections, and establish relationships. This is called pre-training. In post-training, more data and feedback are used to fine-tune the model, often with humans in the loop.
Once a model has been trained, it can be put to the test. This phase is called inference, when the AI answers questions, solves problems, or writes text or code based on a prompt.
Traditionally with AI models, a huge amount of resources goes into training them up front, but relatively fewer resources go towards running them (at least on a per-query basis). DeepSeek did find ways to train its model far more efficiently, both in pre-training and post-training. Advances included clever engineering hacks and new training techniques—like the automation of reinforcement feedback usually handled by people—that impressed experts. This led many to question whether companies would actually need to spend so much building enormous data centers that would gobble up energy.
It’s Costly to Reason
DeepSeek is a new kind of model called a “reasoning” model. Reasoning models begin with a pre-trained model, like GPT-4, and receive further training where they learn to employ “chain-of-thought reasoning” to break a task down into multiple steps. During inference, they test different formulas for getting a correct answer, recognize when they make a mistake, and improve their outputs. It’s a little closer to how humans think—and it takes a lot more time and energy.
In the past, training used the most computing power and thus the most energy, as it entailed processing huge datasets. But once a trained model reached inference, it was simply applying its learned patterns to new data points, which didn’t require as much computing power (relatively).
To an extent, DeepSeek’s R1 reverses this equation. The company made training more efficient, but the way it solves queries and answers prompts guzzles more power than older models. A head-to-head comparison found that DeepSeek used 87 percent more energy than Meta’s non-reasoning Llama 3.3 to answer the same set of prompts. Also, OpenAI—whose o1 model was first out of the gate with reasoning capabilities—found allowing these models more time to “think” results in better answers.
Although reasoning models aren’t necessarily better for everything—they excel at math and coding, for example—their rise may catalyze a shift toward more energy-intensive uses. Even if training models gets more efficient, added computation during inference may cancel out some of the gains.
Assuming that greater efficiency in training will lead to less energy use may not pan out either. Counter-intuitively, greater efficiency and cost-savings in training may simply mean companies go even bigger during that phase, using just as much (or more) energy to get better results.
“The gains in cost efficiency end up entirely devoted to training smarter models, limited only by the company’s financial resources,” wrote Anthropic cofounder Dario Amodei of DeepSeek.
If It Costs Less, We Use More
Microsoft CEO Satya Nadella likewise brought up this tendency, known as the Jevons paradox—the idea that increased efficiency leads to increased use of a resource, ultimately canceling out the efficiency gain—in response to the DeepSeek melee.
If your new car uses half as much gas per mile as your old car, you’re not going to buy less gas; you’re going to take that road trip you’ve been thinking about, and plan another road trip to boot.
The same principle will apply in AI. While reasoning models are relatively energy-intensive now, they likely won’t be forever. Older AI models are vastly more efficient today than when they were first released. We’ll see the same trend with reasoning models; even though they’ll consume more energy in the short run, in the long run they’ll get more efficient. This means it’s likely that over both time frames they’ll use more energy, not less. Inefficient models will gobble up excessive energy first, then increasingly efficient models will proliferate and be used to a far greater extent later on.
As Nadella posted on X, “As AI gets more efficient and accessible, we will see its use skyrocket, turning it into a commodity we just can’t get enough of.”
If You Build It
In light of DeepSeek’s R1 mic drop, should US tech companies be backpedaling on their efforts to ramp up energy supplies? Cancel those contracts for small modular nuclear reactors?
In 2023, data centers accounted for 4.4 percent of total US electricity use. A report published in December—prior to R1’s release—predicted that figure could balloon to as much as 12 percent by 2028. That percentage could shrink due to the training efficiency improvements brought by DeepSeek, which will be widely implemented.
But given the likely proliferation of reasoning models and the energy they use for inference—not to mention later efficiency-driven demand increases—my money’s on data centers hitting that 12 percent, just as analysts predicted before they’d ever heard of DeepSeek.
Tech companies appear to be on the same page. In recent earnings calls, Google, Microsoft, Amazon, and Meta announced they would spend $300 billion—mostly on AI infrastructure—this year alone. There’s still a whole lot of cash, and energy, in AI.
The post DeepSeek Crashed Energy Stocks. Here’s Why It Shouldn’t Have. appeared first on SingularityHub.
* This article was originally published at Singularity Hub
0 Comments